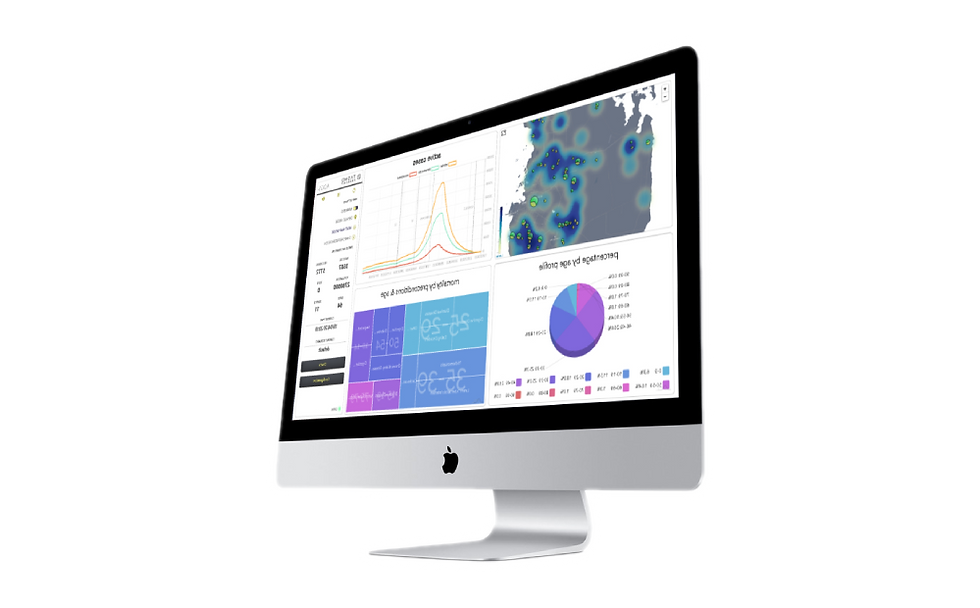
When improving a national or regional pandemic model, PANDEXIT software provides the ability to discover dependencies and quantify the effects of policy change, resulting in the optimal targeting of efforts and prioritization of changes.
Epidemiological research benefits from the ability to model contagion dynamics and social interactions. With epidemic simulation forecasting and scenarios can be tested and evaluated, informing mitigation strategies and increasing preparedness.
Unveiling Pandemic Dependencies for Informed Policy Change
pandexit: the AGENT-BASED MODELING OF COVID-19 PANDEMIC
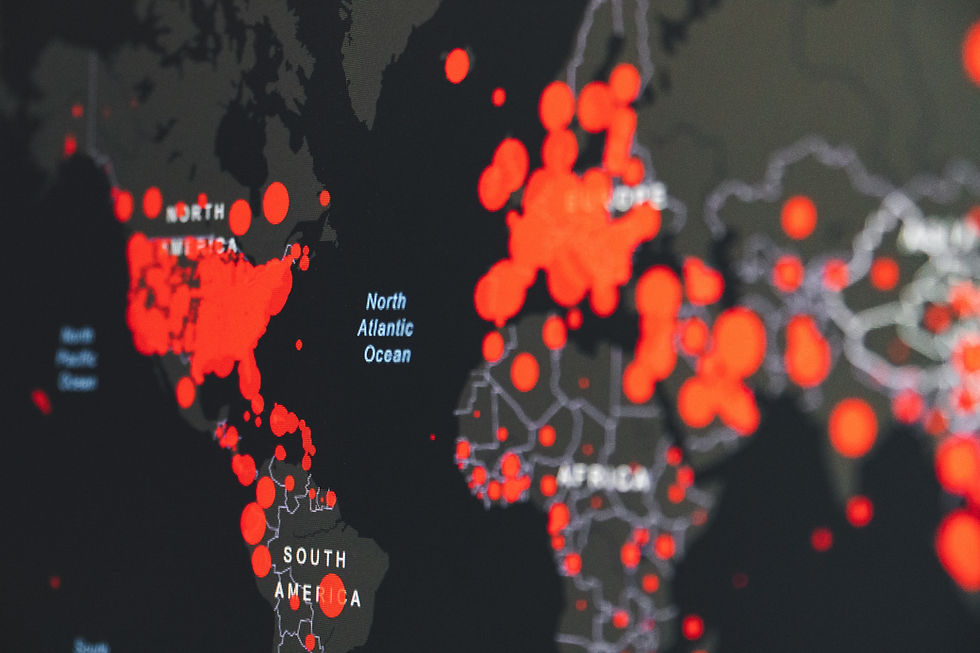
Elevate Decision-Making with PANDEXIT: A Fine-Tuned Pandemic Modeling Tool

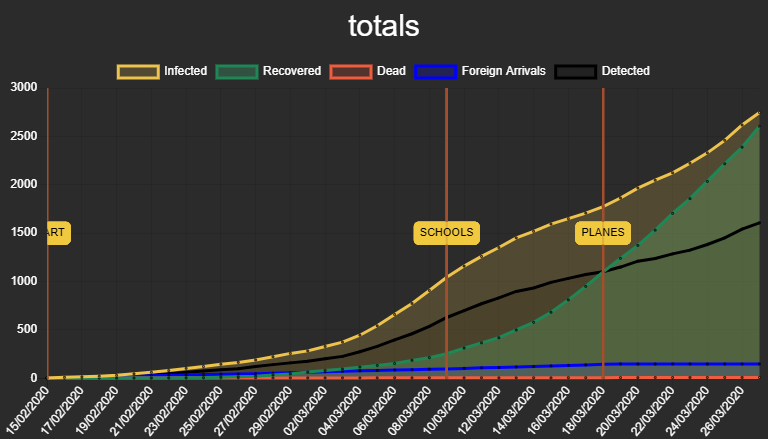
PANDEXIT is a tool to help decision makers evaluate the outcome of different COVID-19 lockdown policies through a reasonable estimation of the evolution of the pandemic in several configurable scenarios.
Specifically, PANDEXIT is an agent-based simulation - a method that has a long-established history in academic research, both for pandemics and economic modelling. It follows every virtual agent (adult or child) through the day, matching its travelling, educational, working, and buying behaviour to the one corresponding to its demographic group, and simulating its interactions to better model the evolution of the pandemic under different lockdown scenarios in a detailed manner – be at a local, regional, and/or national level.
It’s a far superior approach then the commonly used statistical techniques that rely on limited aggregated data, often times after-the-fact analysis, and most importantly ignore individual human interaction points in which viruses thrive.
The level of granularity that is required to provide much more accurate predictions is one important aspect that distinguishes PANDEXIT from other available agent-based models. The other factor is that the algorithm has been fine-tuned over the last 30 years.
Using the PANDEXIT tool will provide a significant pillar of confidence in determining the best strategy in a way that considers the particular demographic, geographic, and economic conditions to avoid the costs of an erroneous approach.
The COVID-19 pandemic has forced a worldwide lockdown and quarantine of suspected cases, which includes restricting the businesses and organizations that are considered essential to remain open, and severely limiting the movement of people inside the country. However, eventually the lockdown and travel restrictions will need to be lifted gradually. That will require unparalleled consideration of public health concerns and minimising catastrophic economic consequences. Which policies to implement, in which order, how to gradually phase them in, and how to consider the peculiar conditions of each territory is not only a sovereign prerogative, but also a problem subject to the particular demographics, geography, political divisions, and economical structure of any country. Encompassing the complex scenarios presented by this novel crisis, a possible second wave of contagion, and future challenges of a related category will require original tools.
Navigating the Uncharted Waters: Balancing Health and Economy in a Post-Pandemic World

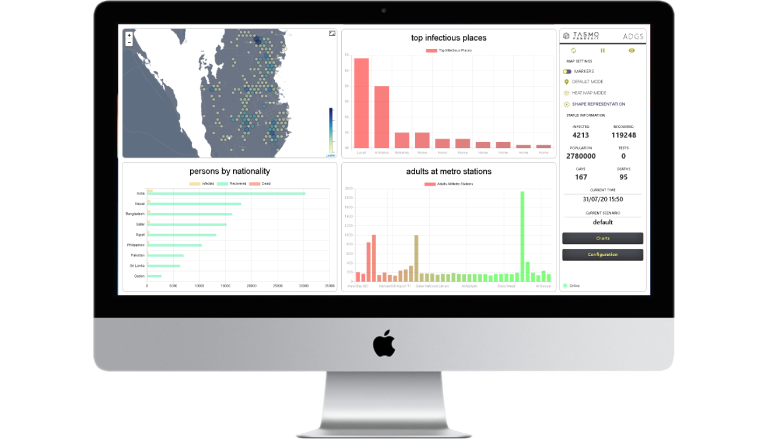
WHAT IS THE PROBLEM WITH THE CURRENT SITUATION?
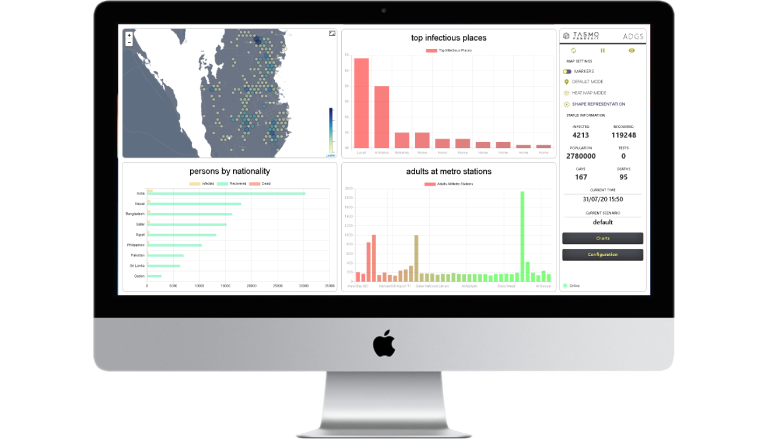
The necessary information for critical decision making during unprecedented crises is generally lacking. Statistical models for previous pandemics might no longer apply due to the natural growth in the country or modified conditions. For instance, the SIR (susceptible, infected, recovered) model is an example of a widely used statistical model, where the three groups of persons and their size, together with their time evolution, is considered in order to describe the evolution of the pandemic. This does not at all consider the fact that the phenomenon of the COVID-19 pandemic is the emergent property of human interaction.

The bottom line is that classic statistical modelling cannot simulate how big data of demographics, behaviour, transportation routes, and work conditions all work together in minute detail at a nationwide level.Subsequently, we have the current situation where determining the best strategy that considers the particular demographic, geographic, and economic conditions of a country to avoid the economical and public health costs of an erroneous approach appears to be remote.
HOW DOES PANDEXIT CONTRIBUTE TO SOLVE THE PROBLEM?
Instead of using statistical techniques to capture the behaviour of relevant groups in an aggregated fashion, we use agent-based modelling that focuses on simulating every individual part of the system under consideration.
Agent-based modelling is a class of computational models for simulating actions and interactions of autonomous agents to assess their emergent effects on a system, i.e. the pandemic, as a whole. Agent-based models are capable of evaluating the outcomes for the specific conditions of a country or a region.
With access to big data describing demographics, behaviour, transportation routes, and work conditions, together with the extensive availability of computational resources, a country-wide simulation can be carried out in minute detail. All the calculated data is then processed by a proprietary Artificial Intelligence engine that delivers human readable information.
The result being that PANDEXIT will support decision makers in evaluating the outcome of different COVID-19 lockdown policies through a reasonable estimation of the evolution of the pandemic in several configurable scenarios.
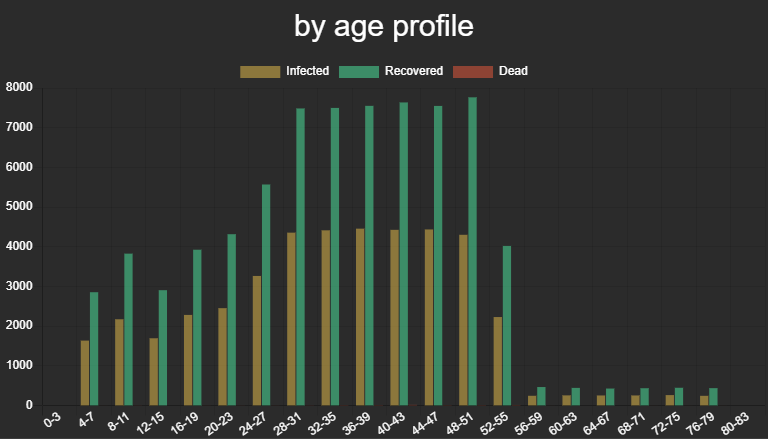
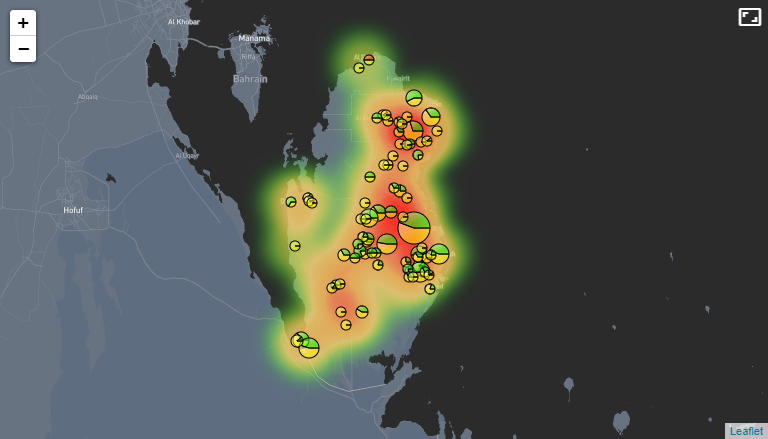
Find out more by watching our videos
NAHUEL GONZALEZ
"THE SAME AS BIOLOGICAL LIFE IS AN EMERGENT PROPERTY OF CHEMISTRY, THE PHENOMENON OF THE COVID-19 PANDEMIC IS THE EMERGENT PROPERTY OF HUMAN INTERACTION"
WHAT IS THE PROBLEM WITH THE CURRENT SITUATION?
Instead of using statistical techniques to capture the behaviour of relevant groups in an aggregated fashion, we use agent-based modelling that focuses on simulating every individual part of the system under consideration.
Agent-based modelling is a class of computational models for simulating actions and interactions of autonomous agents to assess their emergent effects on a system, i.e. the pandemic, as a whole. Agent-based models are capable of evaluating the outcomes for the specific conditions of a country or a region.
With access to big data describing demographics, behaviour, transportation routes, and work conditions, together with the extensive availability of computational resources, a country-wide simulation can be carried out in minute detail. All the calculated data is then processed by a proprietary Artificial Intelligence engine that delivers human readable information.
The result being that PANDEXIT will support decision makers in evaluating the outcome of different COVID-19 lockdown policies through a reasonable estimation of the evolution of the pandemic in several configurable scenarios.
ADGS has been built around a franco-qatari friendship and partnership to create a Deep Tech Company in Qatar, researching and developing Artificial Intelligence applied to human language, Behavioral Biometrics and Emergent Behavior. We could say that the surprising ADGS achievements come from an extraordinary team working in perfect harmony.
PANDEXIT is a tool for helping informed decision makers visualize and understand the possible outcomes of alternative scenarios by simulating, with a realistic agent-based model, the spread of the pandemic inside a country or region under different policies and conditions. PANDEXIT is not a real time tracking system, nor another refined statistical model; it is a detailed one-to-one simulation of every person in the territory under consideration and his or her whereabouts.
Decision making is not about the past. It is about the future, and the future cannot be known with certainty. However, with precise and faithful data, as well as enough computing power, the uncertainty can be reduced, and many initially unexpected outcomes can be discovered and explained. You can see that every day in the weather forecast. It cannot tell you exactly what the temperature will be tomorrow, but you can get a very good approximation which might save you some inconveniencies. PANDEXIT is useful for governments fighting a pandemic as it allows them to estimate the future outcome of policies that are being considered but not yet implemented in a risk-free way, based on the specific characteristics of the territory and not generalizations which might not apply
Inside PANDEXIT, every simulated person is a small program that goes through its daily life as we would in the real world. It wakes up at his home, commutes to his job, goes to the supermarket a few times a week, has friends and family that he visits with a certain frequency, sometimes goes to the church or mosque to follow his religious practices. Every one of these places is represented geographically inside PANDEXIT, and so are the transport means used to reach them by the agent. Millions of these simulated persons move around the virtual world, following (and sometimes defying, or forgetting to enforce) the policies set up by the administrator, such as forcing them to use masks in public, checking temperature at shop entries, restricting travel from some areas to others, etc. Some of these agents are infected and transmit the pandemic around as their real-world counterparts.
An agent-based model is a class of computing models which simulate the actions and interactions of a set of autonomous agents, with the objective of understanding and predicting the behavior of a system as a whole. This stands in contrast to a statistical or dynamic model, where the persons are aggregated in groups like susceptible, infected, and recovered, not considered individually. Specifically, for PANDEXIT, this means every person in the territory under consideration is simulated by a small program that follows his or her daily routine.
The model of PANDEXIT contains three types of entities: persons, places, and transport. Places are characterized by their geographical coordinates, which kind of place it is (as in office, hospital, shopping centre, small shop, church, etc.), and its size. Transports are characterized by their type (car, metro, bus, etc.), their size, and to which line or route they belong. Persons are the most complex entities in the system; they are characterized by their age, nationality, shopping habits, transport strategy, etc. It is also needed to link these three types of entities. Every person has a home, most have a job to attend (or a school if they are children), a preferred set of shops they use frequently, a list of friends they visit with certain frequency, possibly a family, and some social gatherings they enjoy. This is the baseline of data needed, but some of it can be generated using demographic models. For more complex scenarios, obviously more data is needed. Say, if you want to understand how different businesses are affected or contribute to the spread, we will need to separate the places by category.
You do not need to actually do a manual data entry person by person, place by place, transport by transport. The data to feed the simulation can be imported from existing databases the government already has, and the missing information can be estimated with demographic models. Of course, the more actual data representing the real world you can provide, the better the results of the simulation will be.
No, it doesn’t. PANDEXIT is an agentbased simulation tool, not a real time tracker. It is not following you around. Inside the simulation there is an agent that has your age and nationality, lives roughly where you live, and roughly follows your daily routine, but there is no live synchronization between the simulated agent and you. The data required to represent your digital surrogate is very similar to one produced by a census.
Yes, it can, but with a proviso. If you feed the simulation with the aforementioned data for different communities, you can compare the outcome for each of them. The basic reproduction number, R0, that is mentioned everywhere in the news nowadays is not a property of the pandemic per se, but a resultant of the characteristics of the pandemic and the community together. Even if the virus is the same, when infecting a more aware population that can respect and follow accurately the safety guidelines, its basic reproduction number will be much lower than in the presence of generalized risky behavior. PANDEXIT, if fed with enough precise data, can capture these underlying conditions and give a rough prediction of the relative outcomes in different communities. But there is a proviso. We are talking here about the comparison of relative outcomes, not scenarios valid in the absolute sense. There are currently too many uncertainties for anybody to claim absolute accuracy. If PANDEXIT predicts 5000 deaths in a community that does not mean that will not be 4900 or 5100, but if it predicts double that number for another one, it is surely at much higher risk. As an example of modeling specific communities, our first prototype was commissioned by the Ministry of Defense of Argentina to evaluate the spread inside the armed forces and the military schools.
No, it cannot. Nobody can, and you should not trust anybody saying they can. There are currently too many uncertainties for anybody to claim absolute accuracy. Models, whether statistical, dynamic, agent-based, or of any other kind, are tools for decision making and not crystal balls where you can behold an immutable future. Changes of policy or behavior can make a huge difference, as you can see by comparing how different countries are being affected. That being said, the prediction of the future cases that PANDEXIT generates can be an excellent indicator of relative outcomes, as if a certain community, nationality, economic sector, or region, is more at risk than another.
PANDEXIT can predict subsequent waves of the pandemic arising from policy changes that are premature or mistaken in its aims, or from changes in people’s behavior as in massive protests or disobedience of established health policies. PANDEXIT cannot predict subsequent waves that happen because the virus has mutated or evolved. Once again, nobody can, and you should not trust anybody saying they do. The best scientists can do is to keep track of potential menaces, as in the G4 flu strain discovered a few days ago in China, and set up a system of early warnings.
PANDEXIT can display the evolution of the simulation and of the different configured scenarios in a map of the territory under consideration. In the initial stages each carrier can be tracked individually, and when there are too many the infection can be tracked per group or per zone. The simulation is always done on an individual basis, but for visualization it does not make much sense to show one hundred thousand dots in a map. So, the answer is yes, PANDEXIT can locate the infection foci on a map of the territory, and even attribute to a specific place, as in an industry or an airport. But don’t forget we are talking about the infection foci inside the simulation, not in the real world.
Yes, it only needs to be fed with much more detail on the geometry of the place and interactions inside the building. That would be a separate simulation for a specific building, not a part of the countrywide or regionwide simulation. There is simply not enough processing power available to reach such a level of detail for every building in a country
How fast PANDEXIT is depends on two parameters: which hardware it is running on, and how many entities and places are included in the simulation. Better hardware will obviously give better performance, up to a linear factor, but there is a limit to how much speedup you can gain in this way. The speed scales slightly sublinearly with the number of entities; in simple terms, this means that doubling the number of entities will make the time it takes to complete a simulation increase to something more than double. For example, in an up to date high end server we are running a six months simulation for Qatar, with its population of 2.8 million, in about ten minutes. We are working on a prototype for the whole country of Argentina, with 50 million people, that is expected to take one or two minutes per day of simulation using the same processing power
The only thing it shares is that all are models! In statistical models you consider only groups of people (like susceptibles, infected, recovered, dead, etc.) and the “speed” (we are talking about derivatives, for the informed reader) at which each group spills into the other, which is regulated by a set of differential equations. In an agent-based model you consider every individual person and the conditions of his interactions to track the spread case by case. It is much more computationally expensive while requiring more parameters and input data, but it can show light on complex or specific scenarios that the statistical models cannot apprehend. I am not saying one is better than the other; both have their strengths and weaknesses and are fitted to answer different questions in different time frames. PANDEXIT model offers a number of other specificities not shared with statistical models: - PANDEXIT graphically shows the data using a geographic information system (GIS) - PANDEXIT takes the "small-world" effect into account https://en.wikipedia.org/wiki/Smallworld_network) - PANDEXIT takes places and transports into account and treat them individually, which a statistical model does not consider - PANDEXIT can change any parameter of the simulation while it runs
As with any other infectious disease, you must distinguish the symptom severity and the viral load. The viral load, to put it in simple terms, is how many virii you are carrying and is directly related to how much you can spread it to those around you. However, that does not necessarily correlate with your symptoms’ severity; you could be very sick and show little symptoms, or have a mild infection yet present with high fever. While the spread depends on the viral load, the ability to detect it without testing depends on the symptom severity. PANDEXIT has integrated this consideration in the model, and the administrator can choose whether to allow asymptomatic and oversymptomatic, at which rate they appear in the simulated population, and several other parameters to better capture their influence.
The infection model of PANDEXIT is not rigid. It allows for the administrator to define a set of parameters to better correlate it with current knowledge about the virus characteristics. You can change the infection rate, how much it affects older people in comparison with younger people, the death rate when at the hospital or unaided, whether reinfections are possible or not, whether natural and acquired immunity exists and in which percentage of the population, whether immunity fades away and at which rate, to name some of the parameters the PANDEXIT model supports as it stands. We try to keep the values of those parameters in line with the current scientific consensus, but the administrator is free to change them if there is interest in evaluating an alternative scenario. Thus, if the virus mutates, PANDEXIT can simulate it too. What is more, this makes the model not restricted to coronavirus only
Yes, we have. We are proud to say the comparison of relative outcomes has been accurate, even in view of the remaining uncertainty.
Although a very small number of major countries run agent-based simulations on supercomputers (the TeraGrid in the U.S.), these are more experiments from laboratories and universities than developed and stable products like PANDEXIT. The algorithms at the core of PANDEXIT are so efficient that the requested computing power is far less than these experiments, the costs much lower while the simulation are much easier to setup. Nevertheless, output is equivalent.
Argentina has been the first country to use PANDEXIT. We have been very recently approached by governments interested to prevent or control a second wave. We can name Singapore, Australia, South Africa and even the Navajo Nation. PM Scott Morrison of Australia explained in "The Guardian" how agent-based modeling and I quote "saved Australia from catastrophe"
Although this is a team work, Nahuel Gonzalez is without doubt the main arquitect of PANDEXIT. He is a brilliant Engineer and Researcher on distributed systems, Emergent Behaviour applied to Biology and Human Sciences, Security and Biometrics. He worked with national security agencies, and is a specialist in Artificial Intelligence and Big Data.